About this webinar
​There are many ways to serve ML models to end users today, and even though new ways keep popping up as time passes, many questions remain. Firstly, how do we pick the appropriate serving approach and, secondly, how can we execute it as fast and efficiently as possible?
In this talk, Ramon Perez, our Developer Advocate at Seldon, will dive into the different machine learning deployment strategies available today for both traditional ML systems and Large Language Models, and we’ll also touch on a few dos and don’ts while we’re at it.
Seldon enables a data-centric approach to model inference with ML-focused pipelines and empowers users to run multi-model serving (MMS) strategies. MMS provides a scalable and cost-effective solution when the number of models you are deploying increases.
Both hosting costs and deployment overhead are significantly reduced when using an MMS approach. This is the ideal solution for hosting a large number of models with the same ML framework, or different ones, on a shared platform.
Speakers
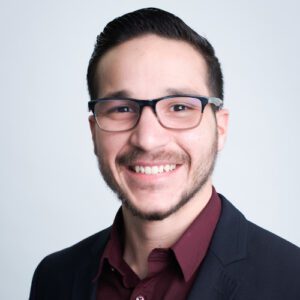
Ramon Perez
What you'll learn
- The different ML deployment strategies on offer
- The best techniques for traditional ML systems and Large Language Models
- How to create a data-centric approach to model inference
- How to scale whilst being cost-effective when creating ML pipelines