In the rapidly evolving field of artificial intelligence (AI), keeping up to date on the advancements and trends is essential for businesses aiming to harness AI’s full potential. We believe, the Gartner Hype Cycle for Artificial Intelligence is a crucial resource for understanding the maturity and adoption of AI technologies. “This research helps AI leaders identify other techniques worthy of investment by profiling a wide range of AI innovations, many of which are advancing fast.”
What is the Gartner Hype Cycle report?
In our opinion, the Gartner Hype Cycle is based on thorough research and direct engagement from analysts and thousands of industry leaders on both sides of the market (buyers and vendors). We believe it serves multiple purposes:
- Technology Evaluation: To help businesses identify which technologies are at the peak of inflated expectations and which are approaching the plateau of productivity.
- Investment Guidance: By understanding where categories sit on the Hype Cycle, companies can make informed decisions about where to allocate resources.
- Trend Analysis: To provide a snapshot of the current state of technology, helping businesses stay ahead of trends and avoid investing in over-hyped but underperforming technologies.
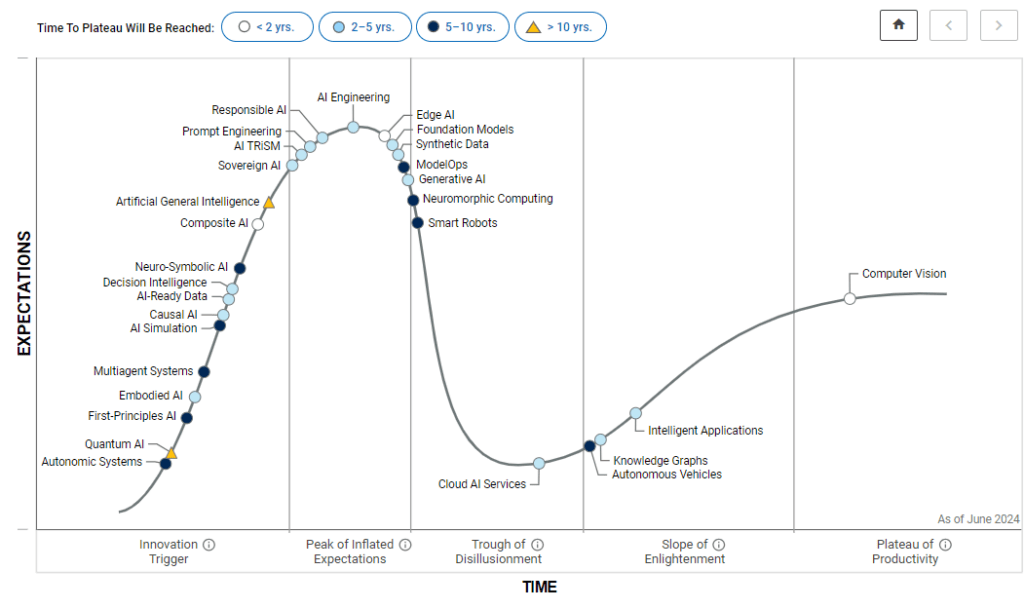
AI Engineering in the Hype Cycle
According to the report, “AI engineering is the foundation for enterprise delivery of AI and generative AI (GenAI) solutions at scale. The discipline unifies DataOps, MLOps and DevOps pipelines to create coherent enterprise development, delivery (hybrid, multicloud, edge), and operational (streaming, batch) AI-based systems.”
As mentioned in the report, the following are the drivers for AI Engineering:
DataOps, ModelOps and DevOps provide best practices for moving artifacts through the AI development life cycle. Standardization across data and model pipelines accelerates the delivery of AI solutions.
The elimination of traditional siloed approaches to data management and AI engineering doubles the data engineering effort and reduces impedance mismatches across data ingestion, processing, model engineering and deployment, which inevitably drift once the AI models are in production.
AI engineering enables discoverable, composable and reusable AI artifacts (data catalogs, knowledge graphs, code repositories, reference architectures, feature stores, model stores and others) across the enterprise context. These are essential for scaling AI enterprise wide.
AI engineering makes it possible to orchestrate solutions across hybrid, multicloud, edge AI or Internet of Things.
Broader use of foundational platforms provides initial success at scaling the production of AI initiatives with existing data, analytics and governance frameworks.
AI engineering practices, processes and tools must be adapted to address GenAI. GenAI specific adaptations include support for prompt engineering, vector DBs/graph KBs, architecting and deploying multiagent, and interactive deployment models.
AI engineering tools can be subdivided into model-centric and data-centric tools. Terms such as DataOps, LLMOps, LangOps or FMOps, or more broader terms such as ModelOps or MLOps, are used frequently, but we believe they are all a subset of AI engineering.
We believe, the placement of AI Engineering at the peak of the Hype Cycle serves as a strong signal that there is significant investment in MLOps platforms from early adopters. In our opinion, this group will be among the first to reach the Plateau of Productivity and realize the enormous potential for value creation from their AI investments.
Seldon Technologies’ Inclusion as a Sample Vendor
Seldon is recognized as a Sample Vendor in the AI Engineering category in the Hype Cycle report. For us, this inclusion is a testament to our innovations and contributions to the AI landscape, particularly in MLOps.
Key products and contributions of Seldon:
- Open Source Tools
○ MLServer: A lightweight inference server designed to serve machine learning models. It provides an efficient way to deploy models across different environments, ensuring they operate at peak performance.
- Source Available Tools:
○ Seldon Core: A software framework that helps deploy, scale and manage thousands of machine learning models on Kubernetes. Seldon Core facilitates model monitoring, logging, and scaling, which are essential for robust AI engineering practices.
○ Alibi: A Python library developed by Seldon, Alibi provides tools for model interpretability and explainability, addressing critical aspects of AI governance and trust.
- Enterprise Solutions:
○ Seldon Core+: An enhanced version of Seldon Core which offers additional enterprise-grade support and warranties for peace of mind. Core+ can be tailored to meet the needs of larger organizations with more complex requirements.
○ Enterprise Platform: A platform to operationalize models at scale, with seamless governance, risk and compliance management. It includes features like model versioning, A/B testing, and governance, ensuring models are reliable and compliant with regulatory standards.
- Collaborations and Integrations:
○ Seldon’s solutions integrate seamlessly with major cloud providers and AI platforms like Google, AWS and Azure. This compatibility enhances utility in diverse deployment environments, aligning with the AI engineering goal of supporting hybrid and multicloud solutions.
- Community and Ecosystem:
○ Seldon actively contributes to the AI and ML communities, fostering a collaborative environment for developing new features and improvements. This engagement ensures that Seldon’s tools evolve to meet the changing needs of the industry. Seldon also has its own MLOps Community serving users of MLServer, Core and Alibi.
Our Final Thoughts
We believe, Seldon Technologies’s recognition as a Sample Vendor in AI Engineering category in the Gartner report validates our operational excellence in AI. For us, Seldon’s innovative solutions exemplify the best practices in deploying and managing AI models at scale, making us a valuable partner for enterprises navigating the complex AI landscape. As AI continues to transform industries, our role in enabling scalable, reliable and explainable AI will be increasingly vital. We think, by following the Gartner insights and leveraging the strengths of Seldon, businesses can effectively integrate AI Engineering into their operations, driving significant value and staying ahead in the competitive market.
GARTNER is a registered trademark and service mark and HYPE CYCLE is a trademark of Gartner, Inc. and/or its affiliates in the U.S. and internationally and are used herein with permission. All rights reserved. Gartner does not endorse any vendor, product or service depicted in its research publications, and does not advise technology users to select only those vendors with the highest ratings or other designation. Gartner research publications consist of the opinions of Gartner’s research organization and should not be construed as statements of fact. Gartner disclaims all warranties, expressed or implied, with respect to this research, including any warranties of merchantability or fitness for a particular purpose.