Enable your MLOps team with two powerful source available Python libraries for post-deployment monitoring to ensure better reliability in your applications.
Alibi Detect helps ensure confidence with performance monitoring
Alibi Explain helps teams gain ‌richer insights with model explainers and predictions
MLOps shouldn't stop at deployment
Make your machine learning efforts more reliable and build confidence in your deployed models with tools like enhanced outlier, adversarial and drift detection.
Take control of model performance with advanced drift detection
Notice changes in data dynamics & define whether detected drift will cause a decrease in model performance.
Discover critical anomalies in input and output data using outlier detection
Alert business units and users when seeing unexpected behavior.
Adversarial detection ensures that models perform consistently
Return the score that indicates the presence of features & instances that trick the model outcome.
Start Now
Start using Alibi Detect today through GitHub. You’ll only need a license for production use. It’s free for all non-production and academic uses.Â
Features
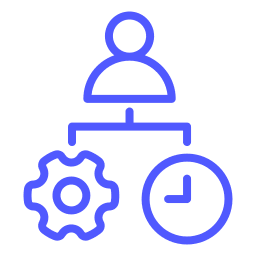
Workflows
Front-end deployment of models, explainers and canaries means non-Kubernetes experts can deploy ML models and testing can be done in live environments.
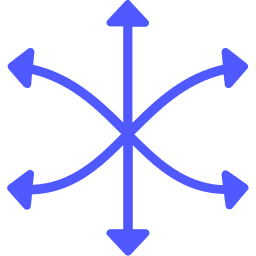
Model management
Metrics and dashboards can monitor models to improve performance and rapidly communicate errors for easy debugging.
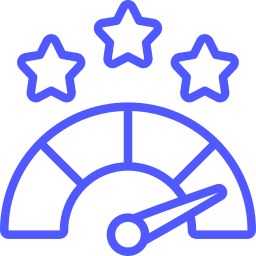
Model confidence
Model explainers mean you can understand and adjust what features are influencing the model and anomaly detection can flag drifts in data and alert users to adversarial attacks.
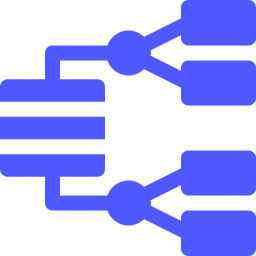
Stack stability
Backwards compatibility, rolling updates and full SLA alongside maintained integrations with all frameworks and clouds means a seamless install and reliable infrastructure.
MLOps shouldn't stop at deployment
Get the most out of your deployed models with added transparency and control over model decisions, fostering trust and understanding through clear explanations for predictions.
Ensure stability of model performance
When your data changes so can your models predictions. Ensure accuracy by monitoring alteration.
Strengthen intuition for feature selection
Gain insights into how features influence model performance.
Derive a set of features and attributes for consistent prediction
Return the score that indicates the presence of features & instances that trick the model outcome.
Start Now
Start using Alibi Explain today through GitHub. You’ll only need a license for production use. It’s free for all non-production and academic uses.Â
Highlights
Feature Alteration
See how prediction changes and ensure stability of model performance against changing data.
Feature Impact
Alibi indicates how features influence model performance, strengthening intuition for feature selection.
Necessary Features
Focus on critical data attributes and features by deriving a set of features and attributes for consistent prediction.
Feature Attribution
Build confidence in integrity of model performance when Alibi illustrates prediction dynamics when features change
Maximize the potential of your MLOps
Ready to put to put Alibi’s Python libraries to work? Any in-production use of Alibi requires the purchase of a business source license.
Alibi is available to purchase with credit card or invoice for production access to the entire Alibi Detect and Explain Python library.Â
£15,000 / €17,250 / $18,000 paid annually
Join our Community
Meet MLOps experts, access support, and stay ahead of the AI curve.
Try before you buy
Explore how Alibi will improve your MLOps projects for free before putting anything into production.